class: center, middle, inverse, title-slide # Single cell protein and RNA analysis ## How can protein help single cell RNA-seq analysis ###
Johan Reimegard
17-Oct-2019 ### NBIS, SciLifeLab --- exclude: true count: false <link href="https://fonts.googleapis.com/css?family=Roboto|Source+Sans+Pro:300,400,600|Ubuntu+Mono&subset=latin-ext" rel="stylesheet"> <link rel="stylesheet" href="https://use.fontawesome.com/releases/v5.3.1/css/all.css" integrity="sha384-mzrmE5qonljUremFsqc01SB46JvROS7bZs3IO2EmfFsd15uHvIt+Y8vEf7N7fWAU" crossorigin="anonymous"> <!-- ------------ Only edit title, subtitle & author above this ------------ --> --- name: intro class: spaced ## WHY PROTEINS? --- name: proRNAchar class: spaced ## The first time I was intrigued with proteins ### How can people with three chr 21 be alive and so well? * DNA level 1.5 times higher on chr 21 * RNA level also higher on chr 21 * Protein levels level same as on other chromosomes * Except for proteins that did not belong to protein complexes * Due to faster degradation of proteins that was not part of a complex .pull-left-30[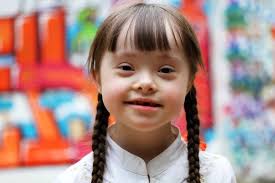] .pull-right-70[ .size-80[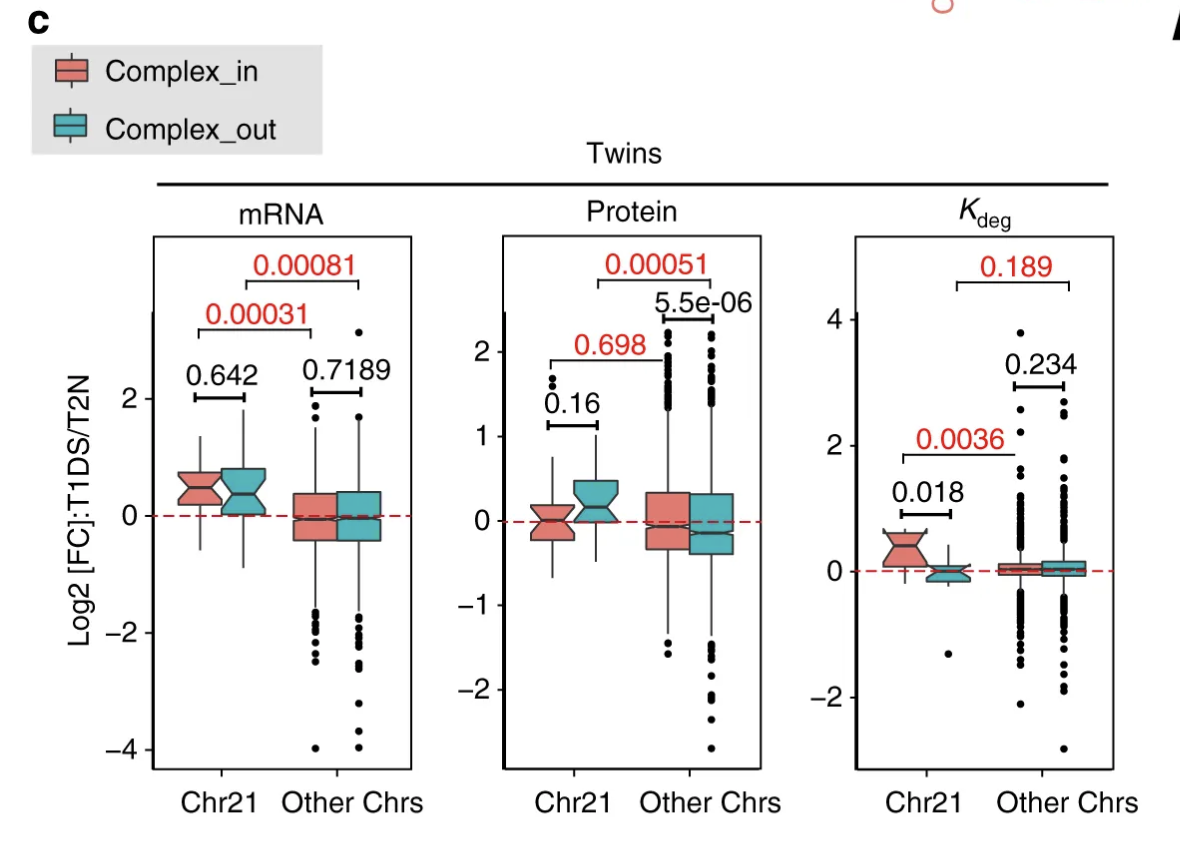] ] .citation[ .cite[<i class="fas fa-link"></i> Stoeckius *et al.* "Systematic proteome and proteostasis profiling in human Trisomy 21 fibroblast cells." [Nature Communications 2017 ](https://www.nature.com/articles/s41467-017-01422-6)] ] --- name: intro class: spaced ## Protein levels better explains the role of a cell than RNA # --- name: intro class: spaced ## Protein levels better explains the role of a cell than RNA # #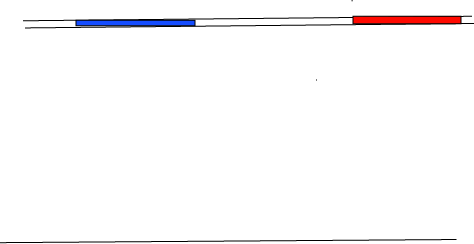 --- name: intro class: spaced ## Protein levels better explains the role of a cell than RNA # #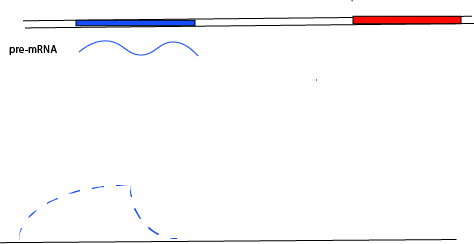 --- name: intro class: spaced ## Protein levels better explains the role of a cell than RNA # #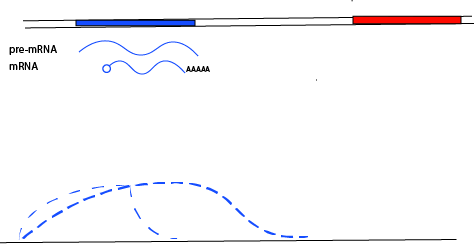 --- name: intro class: spaced ## Protein levels better explains the role of a cell than RNA # #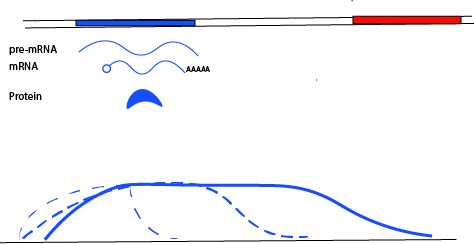 --- name: intro class: spaced ## Protein levels better explains the role of a cell than RNA # #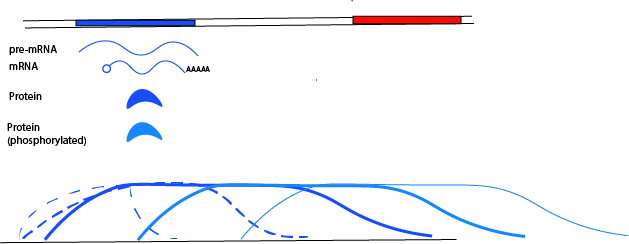 --- name: intro class: spaced ## Protein levels better explains the role of a cell than RNA # #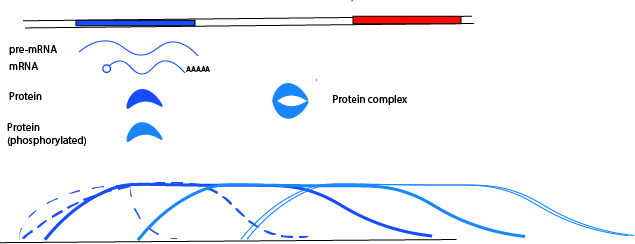 --- name: proAd class: spaced ## Protein levels better explains the role of a cell than RNA # #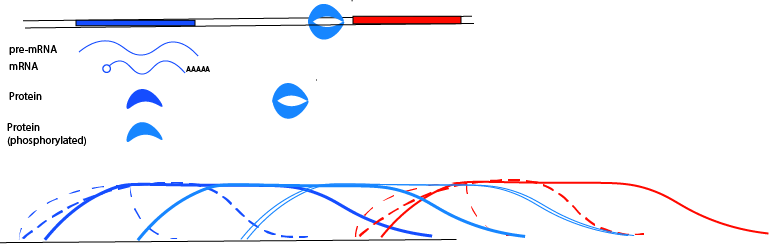 --- name: content class: spaced ## Contents * [WHY PROTEINS](#proRNAchar) * [Protein levels better explains the role of a cell than RNA](#proAd) * [Protein closer to cellular function](#proAd2) * [Protein characteristics](#protCharacteristics) * [Protein cellualar importance](#cellImportance) * [RNA protein dependance ](#ProRNAcorr) * [Post transcriptional modifications](#PostTranscriptMod) * [Single cell protein analysis Landscape](#scpLandscape) * [Single cell protein analysis Trends](#scpTrends) * [PEA and immune assays](#PEA) * [CITE-seq](#CITE) * [SPARC](#CITE) * [Todays afternoon lab](#lab) --- name: protCharacteristics class: spaced ## Protein and RNA characteristics .size-60[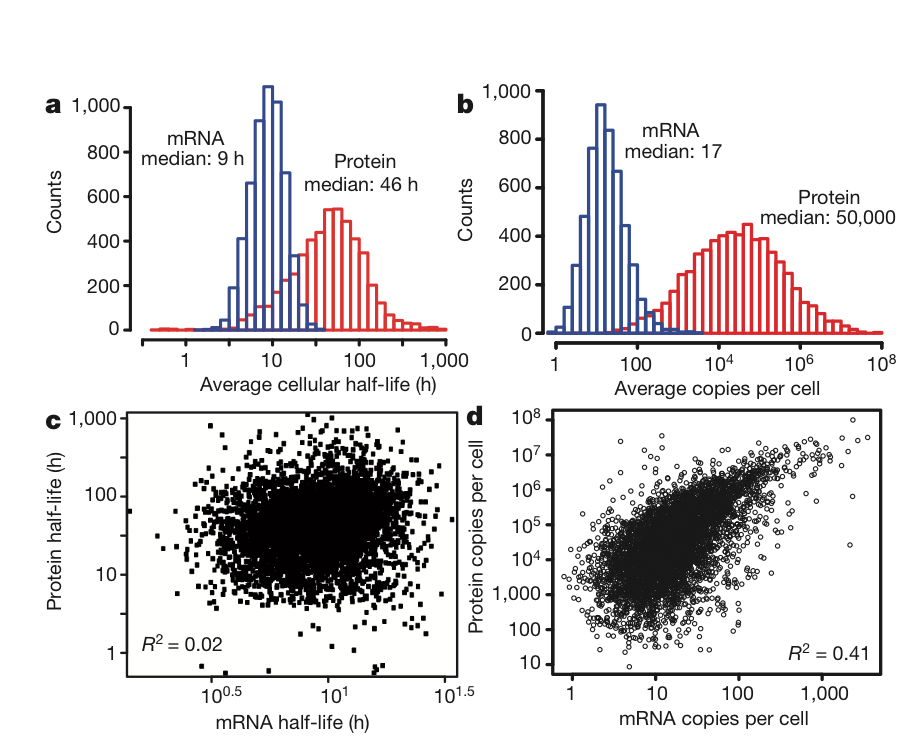] - Proteins are in general more stable - Proteins have a greater dynamic range - Proteins are more abundant .citation[ .cite[<i class="fas fa-link"></i> Schwanh??usser B, *et al.* "Global quantification of mammalian gene expression control" [Nature 2011](https://www.nature.com/articles/nature10098)] ] --- name: ProRNAcorr class: spaced ## Protein and RNA levels does not neccesarily correlate .size-100[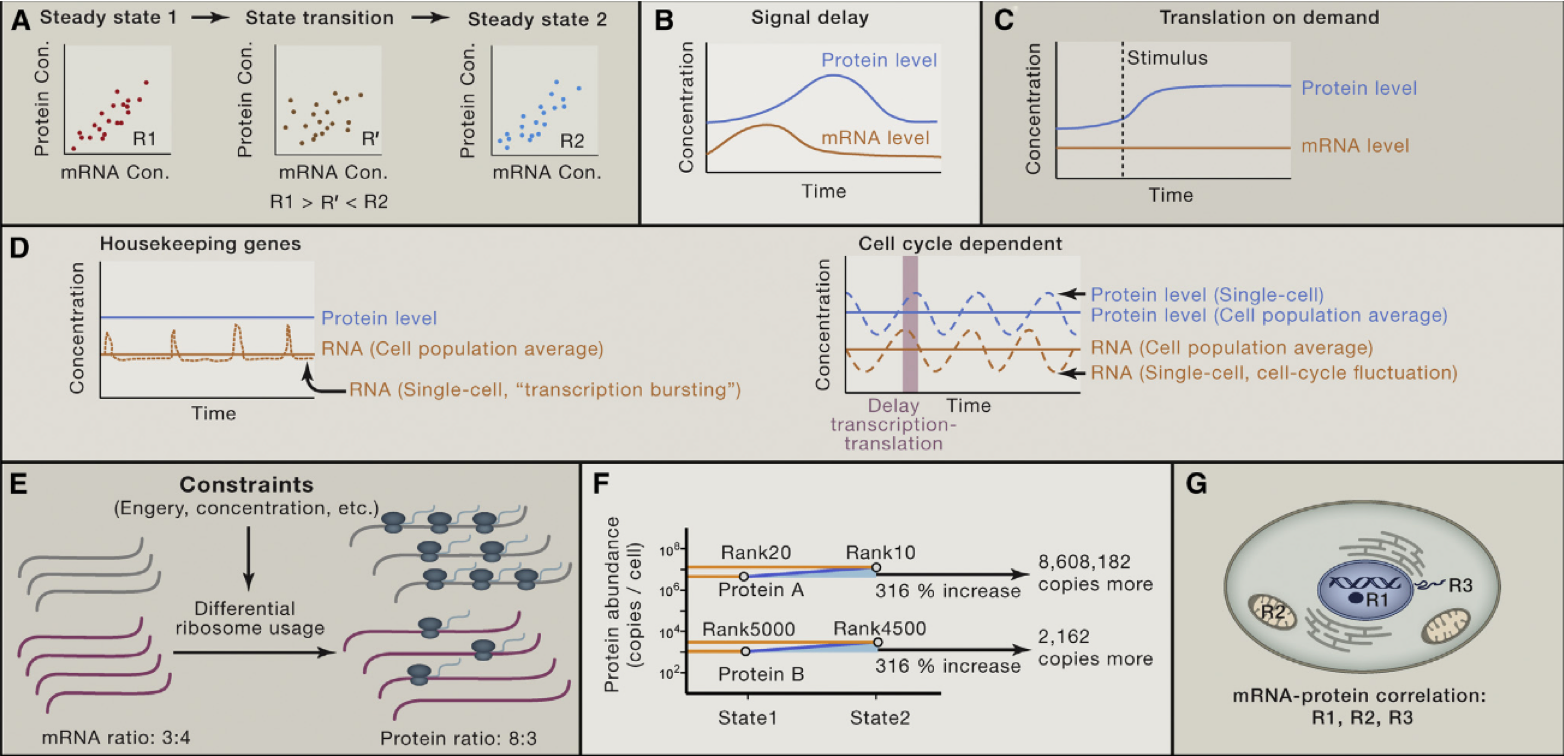] .citation[ .cite[<i class="fas fa-link"></i> Liu, *et al.* "On the Dependency of Cellular Protein Levels on mRNA Abundance" [Cell 165 2016](https://www.cell.com/cell/fulltext/S0092-8674(16)30270-7)] ] --- name: postTransModifications class: spaced ## Post transcriptional modification .pull-left-30[ - Turnover - Protein conformation - Enzyme activity - Cross talk - Localisation - Protein binding and interaction ] .pull-right-70[ .size-85[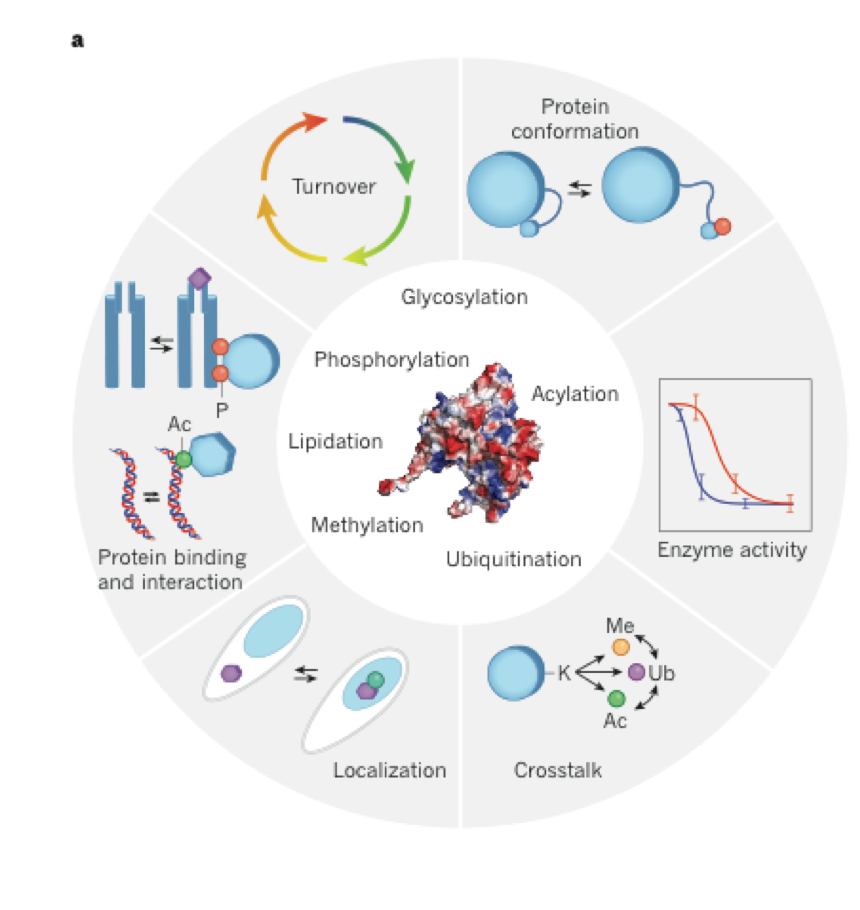] ] .citation[ .cite[<i class="fas fa-link"></i> Aebersold & Mann. "Mass-spectrometric exploration of proteome structure and function." [Nature 2016](https://www.nature.com/articles/nature19949)] .cite[<i class="fas fa-link"></i> See also Wei, *et al*. "Single-cell phosphoproteomics resolves adaptive signaling dynamics and informs targeted combination therapy in glioblastoma." [Cancer Cell. 2016](https://www.ncbi.nlm.nih.gov/pmc/articles/PMC4831071/)] ] --- name: applications class: spaced ## Applications for single cell protein analysis - Biomarkers for cell differentiation - Biomarkers for cell states - Identify post-transcriptional modifications - Identify trans-regulatory targets --- name: scpLandscape class: spaced ## Landscape of single cell protein analysis .pull-left-30[ - Mass spectrometry - Co-detection with RNA - PLA/PEA - ELISA - Immuno-Seq - Immunofluorescence - Mass cytometry - Flow cytometry - Western blot ] .pull-right-70[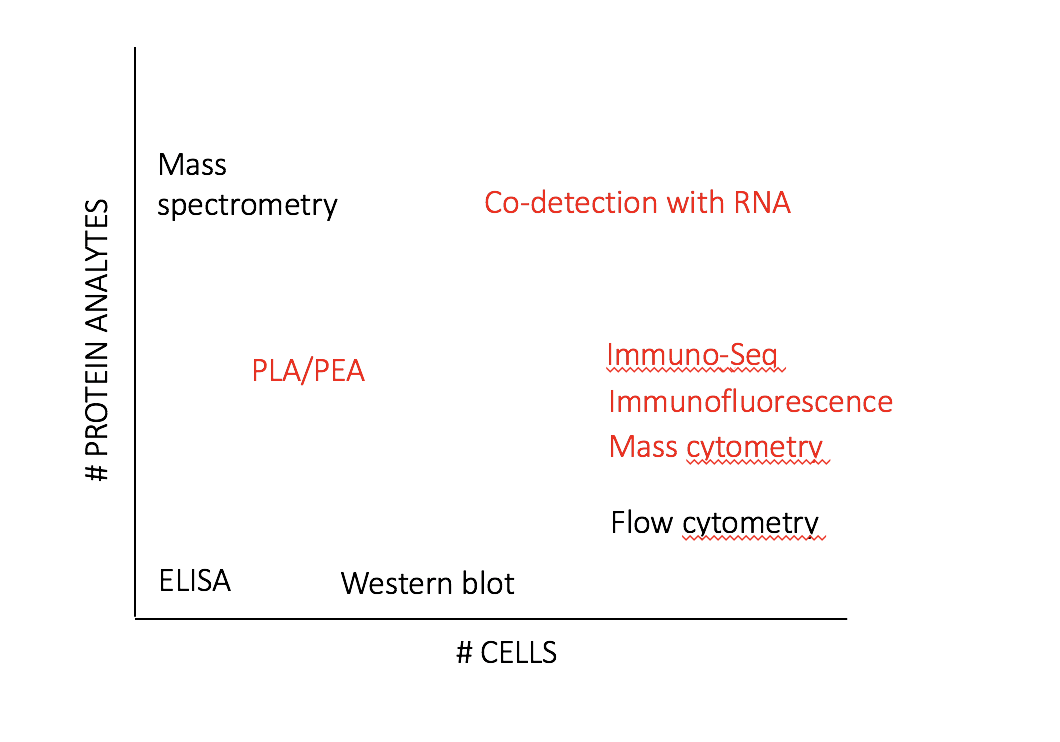 ] --- name: scp_landscape class: spaced ## Landscape of single cell protein analysis .pull-left-50[ **Trends** - Transfer of protein information to DNA - Combined mRNA and protein analysis - Combined in situ multiplex protein and cellular parameters ] --- name: multiIF class: spaced ## Multiplex immunofluorescence .size-90[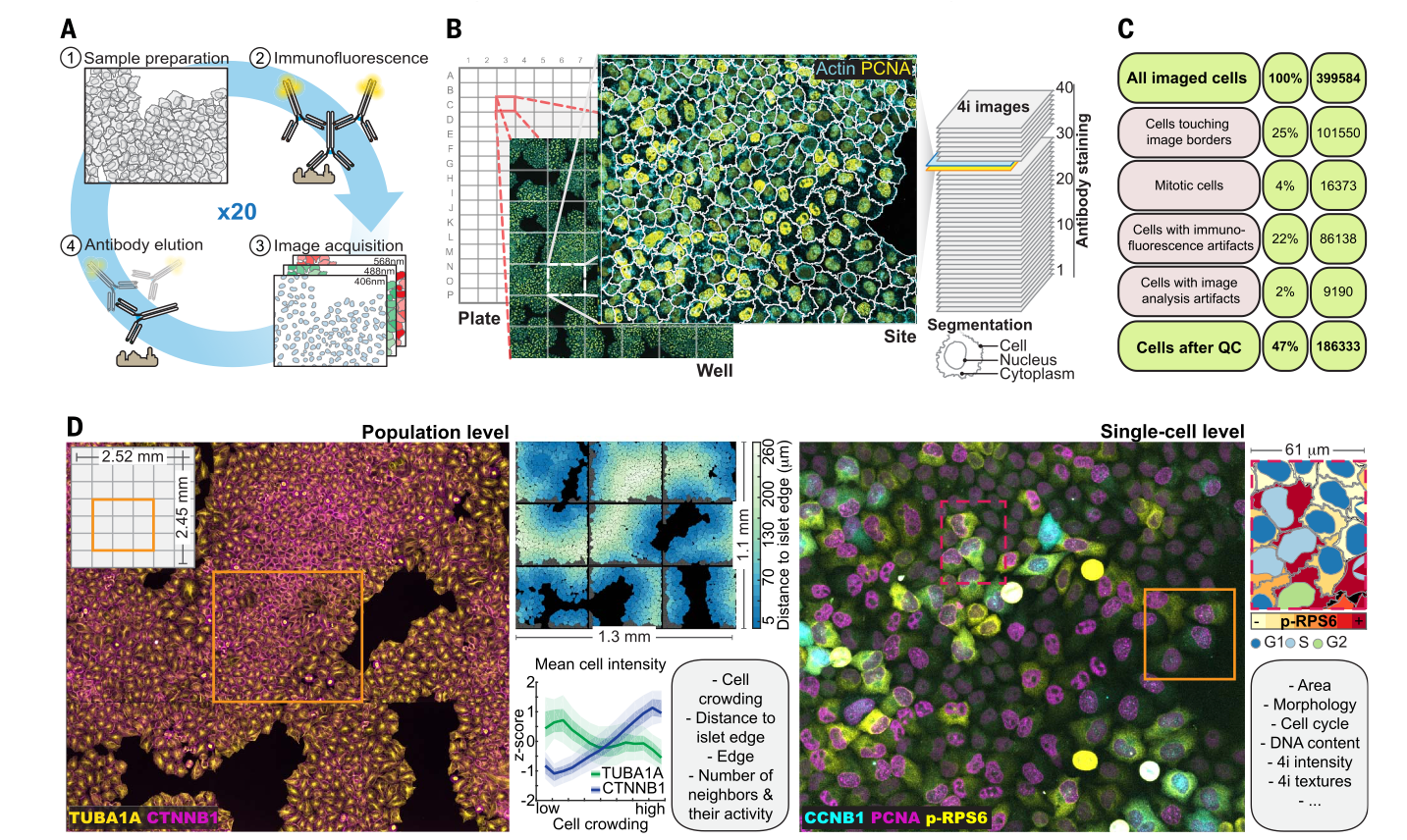] .pull-right-20[ - ~40 plex - Cellular parameters ] .citation[ .cite[<i class="fas fa-link"></i> Gut G *et al.* "Multiplex protein maps link subcellular organization to cellular states." [Science 2018 ](https://science.sciencemag.org/content/361/6401/eaar7042)] ] --- name: MultiProteinDetection1 class: spaced ## Multiplex protein detection Transfer protein information to DNA reporter .pull-left-90[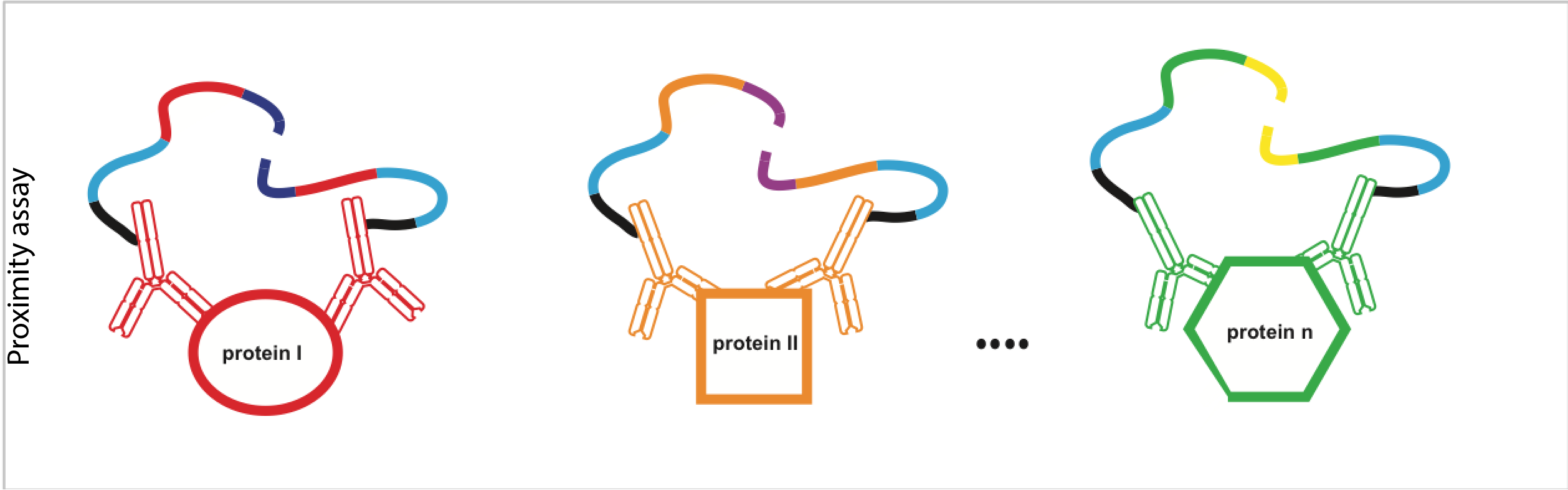] .pull-left-80[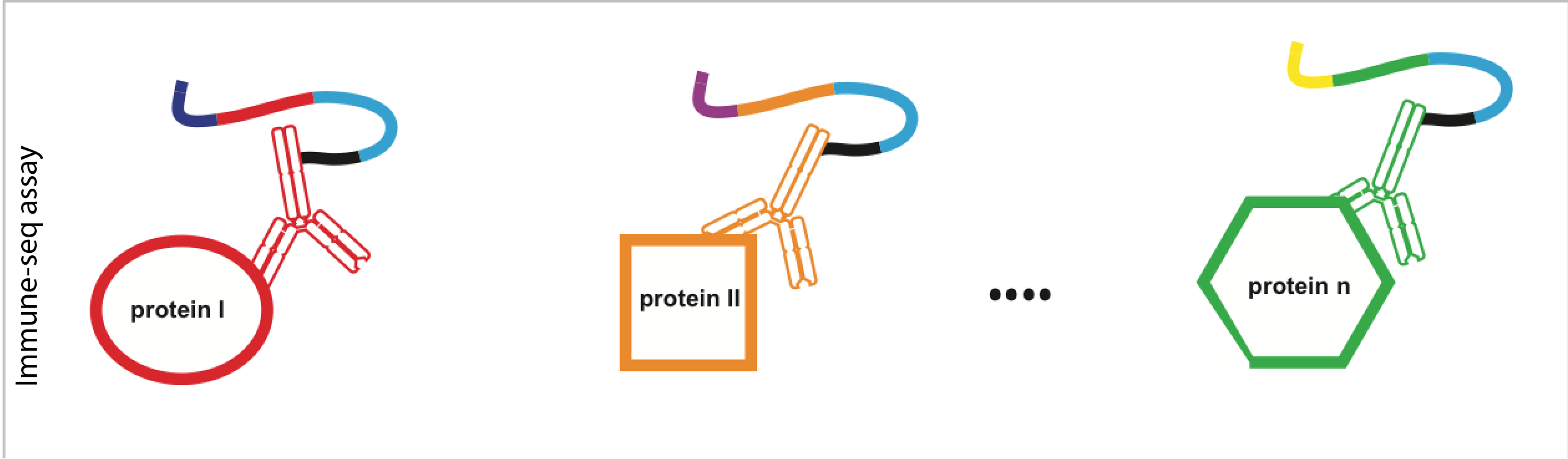] --- name: MultiProteinDetection1 class: spaced ## How does PEA works <iframe width="800" height="400" src="http://www.youtube.com/embed/3-nGHs8DoSw?rel=0" frameborder="0" allowfullscreen></iframe> --- name: MultiProteinDetection2 class: spaced ## Multiplex protein detection .pull-center-50[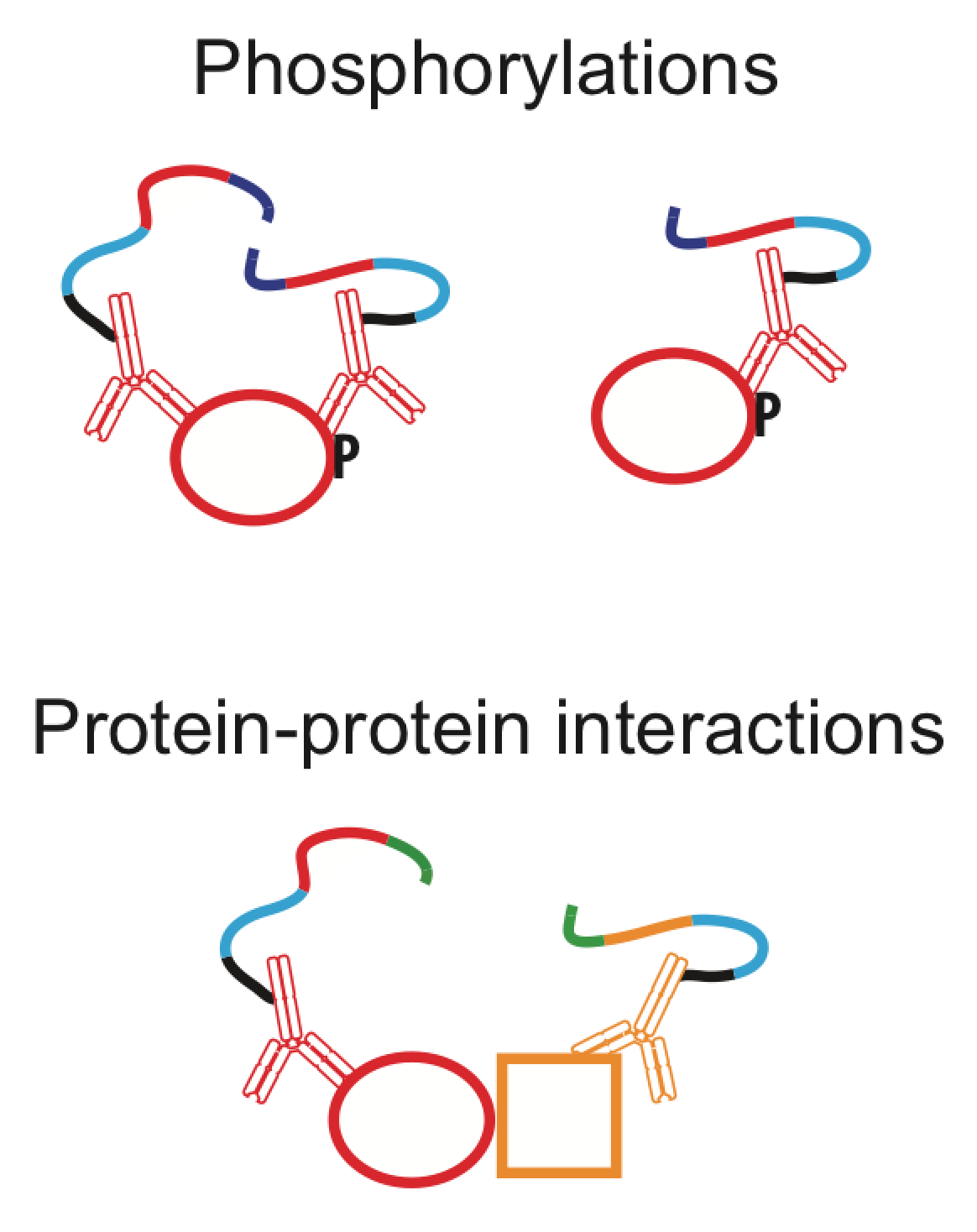] .center[Can also identify more complex scenarios like modification of proteins or protein-protein interactions.] --- name: ImmuneSeq class: spaced ## Immune-Seq Cellular Indexing of Transcriptomes and Epitopes by sequencing (CITE-seq) .pull-center-80[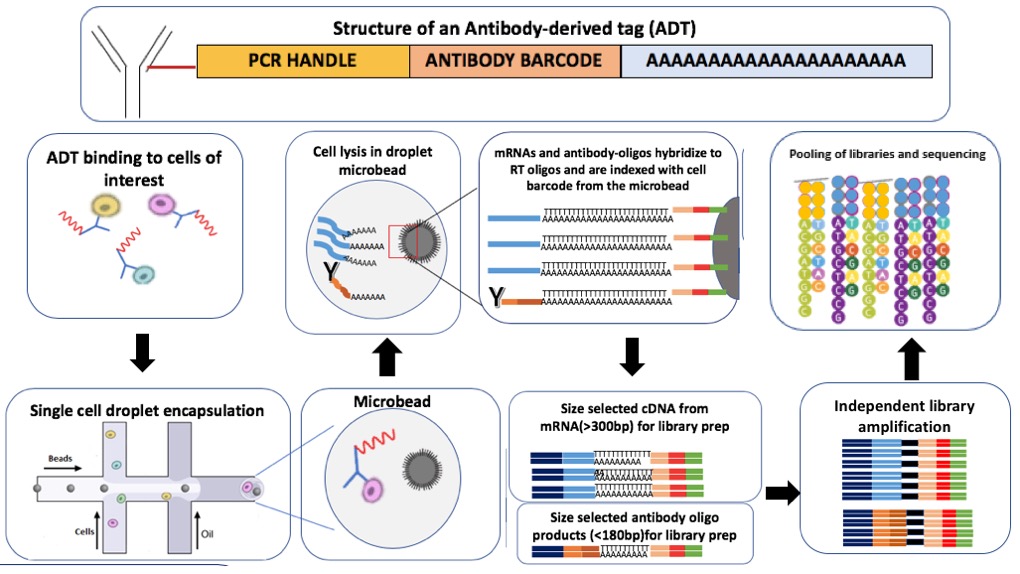] **Disatvantage** Only bind to surface proteins (right now anyway) **Advantage** Can be incorporated in droplet based methods. 10x does CITE-seq. .citation[ .cite[<i class="fas fa-link"></i> Stoeckius *et al.* "Simultaneous epitope and transcriptome measurement in single cells." [Nature Methods 2017 ](https://www.nature.com/articles/nmeth.4380)] .cite[<i class="fas fa-link"></i> Peterson VM *et al.* "Multiplexed quantification of proteins and transcripts in single cells." [Nat Biotech 2017](https://www.nature.com/articles/nbt.3973)] ] --- name: ImmuneSeq class: spaced ## Immune-Seq Can also use it for Cell Hashing .pull-center-80[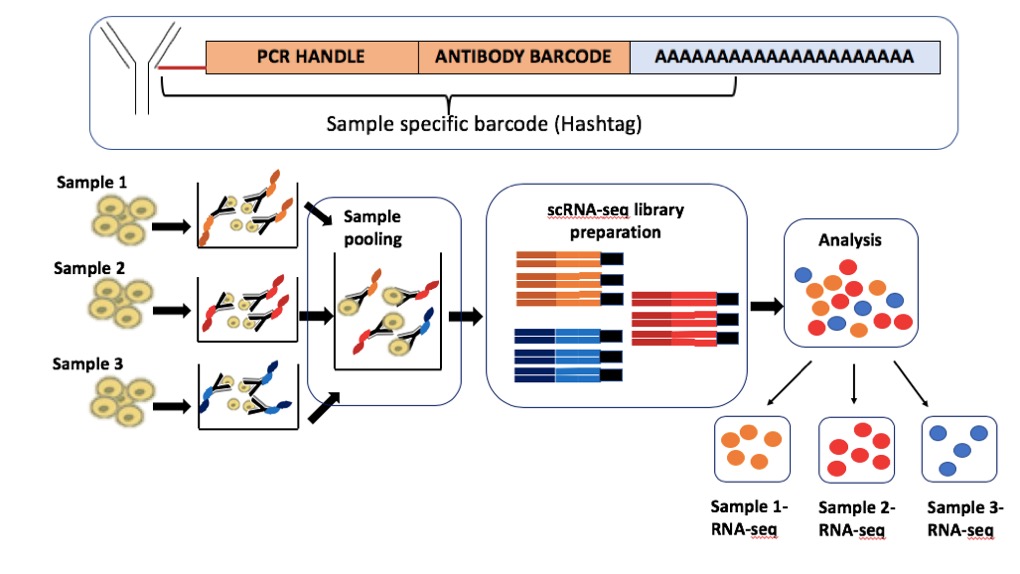] **Advantage** Can join multiple samples before sequencing and use the tags to separate the cells --- name: ImmuneSeq class: spaced ## Immune-Seq ECCITE set published this year from the same group that did CITE-seq. Does scRNAseq, hashtaging, surface proteins and CRISP sequence detection at the same time. .pull-center-80[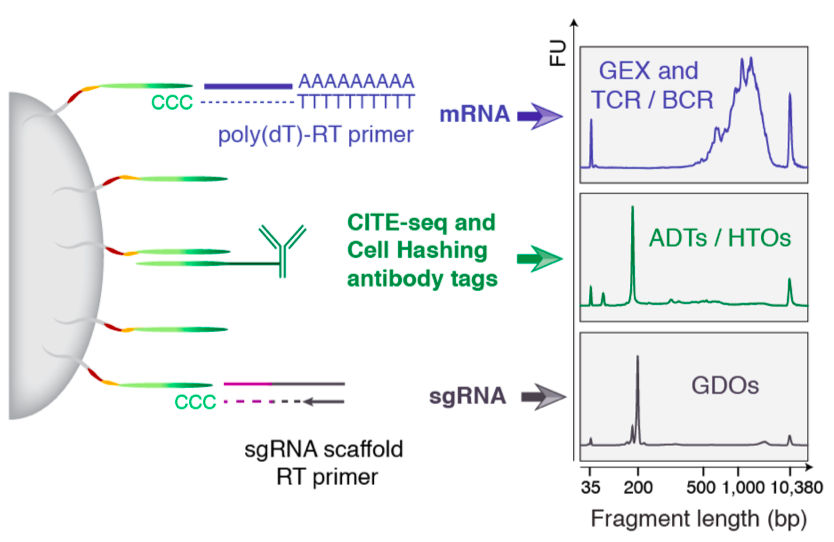] **Advantage** They claim that you can do up to five different assays using this protocol. .citation[ .cite[<i class="fas fa-link"></i> Mimitou EP *et al.* "Multiplex detection of proteins, transcriptomes, clonotypes and CRISPR perturbations in single cells." [Nature Methods 2019 ](https://www.nature.com/articles/s41592-019-0392-0)] ] --- name: ImmuneSeq2 class: spaced ## Protein are stable and make them better biomarkers. * Protein levels in the right context have very long half-life * Especially surface proteins that are very stable #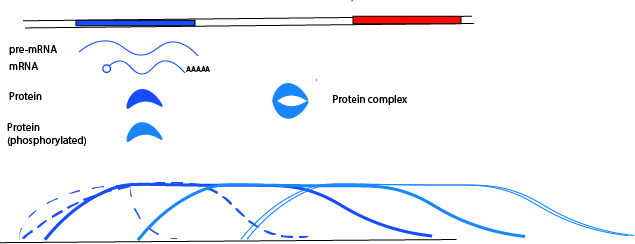 --- name: ImmuneSeq2 class: spaced ## CITE seq experiment on Immune cells. Using the more stable protein expression of surface proteins to distinguish different cell types. .pull-center-80[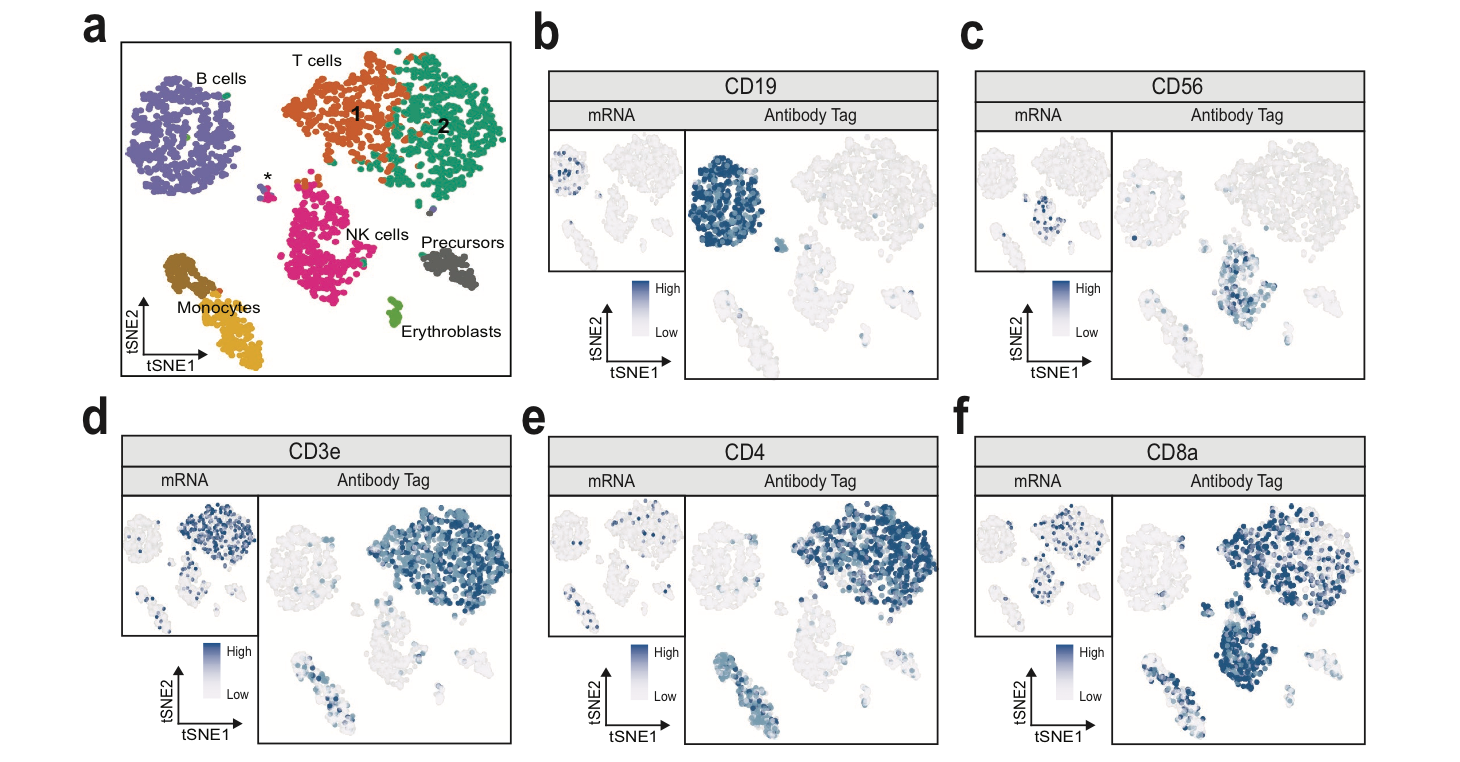] - Surface protein levels per-cells less prone than mRNA to drop-out events (due to greater copy number of proteins?) - Protein data robustly represented immune-phenotypes - scRNAseq data alone could not provide a clear separation between CD4 and CD8 T cells --- name: SPARC1 class: spaced ## Single cell mRNA and protein analysis in a dynamic cellular system .padding-horisontal-70[ - Can we co-measure global mRNA and intracellular protein in single cells? - How are mRNA-protein co-expressed? In cells at steady-state or undergoing a stable-state change? - How can protein measurements aid to decipher cell state and gene regulatory networks? ] --- name: SPARC2 class: spaced ## SPARC - Single-cell Protein and RNA Co-profiling .pull-left-50[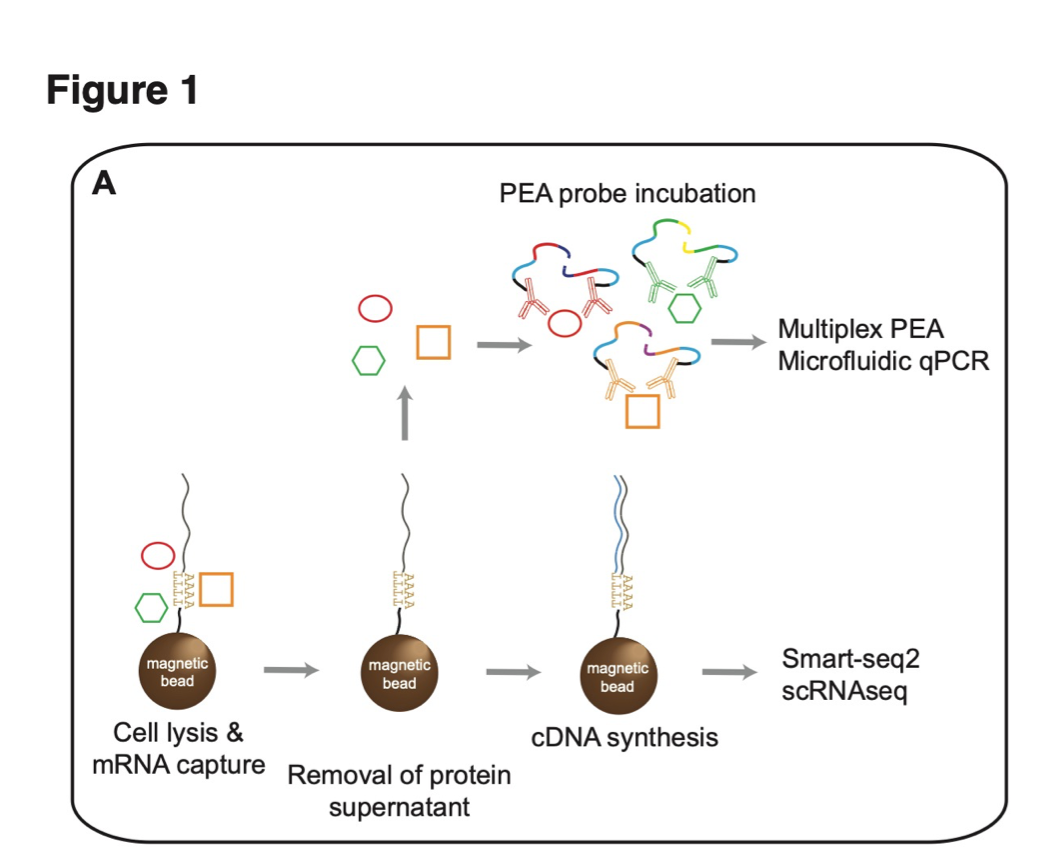] --- name: SPARC2 class: spaced ## SPARC - Single-cell Protein and RNA Co-profiling .pull-left-50[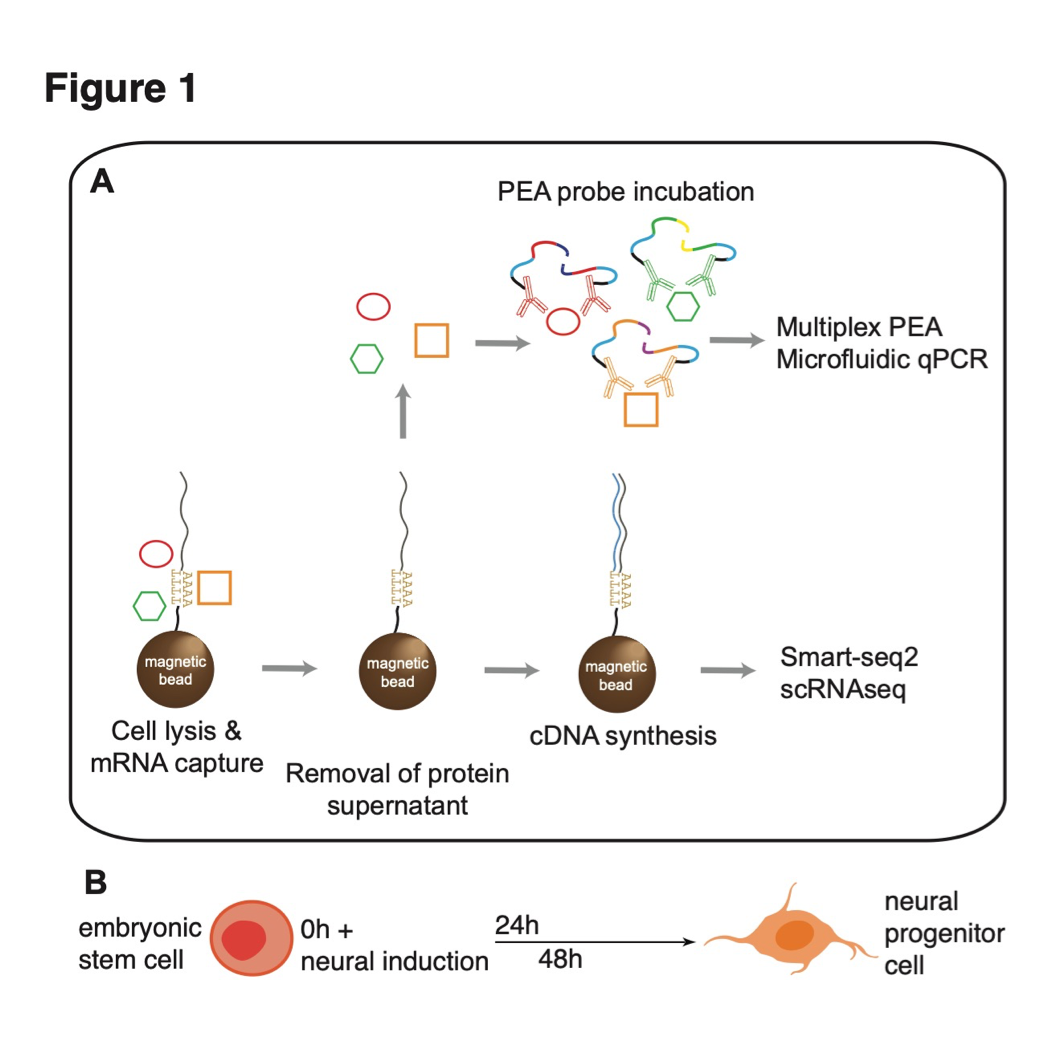] --- name: SPARC2 class: spaced ## SPARC - Single-cell Protein and RNA Co-profiling .size-100[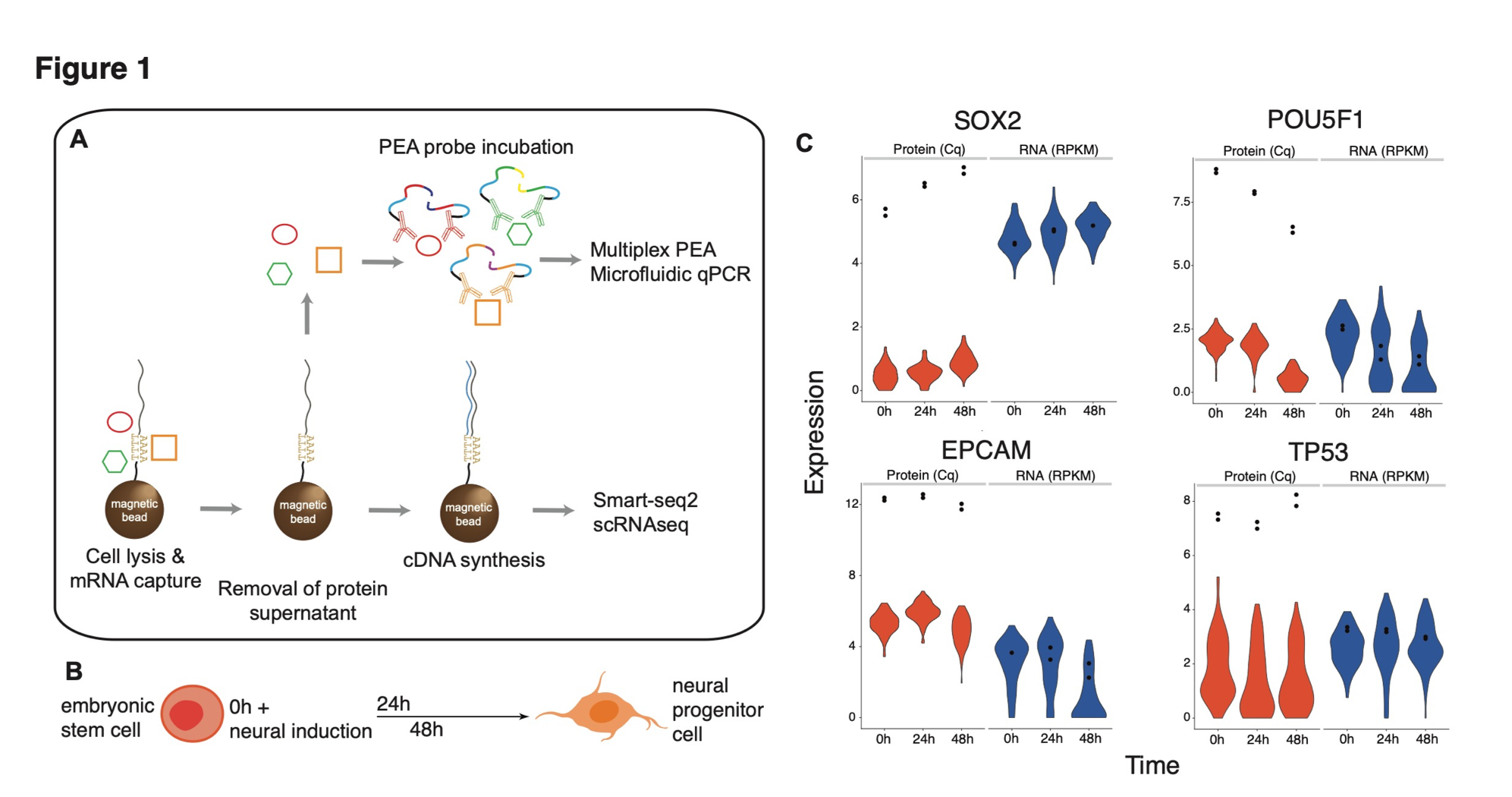] --- name: SPARC2 class: spaced ## SPARC - Single-cell Protein and RNA Co-profiling .size-100[] .pull-left-30[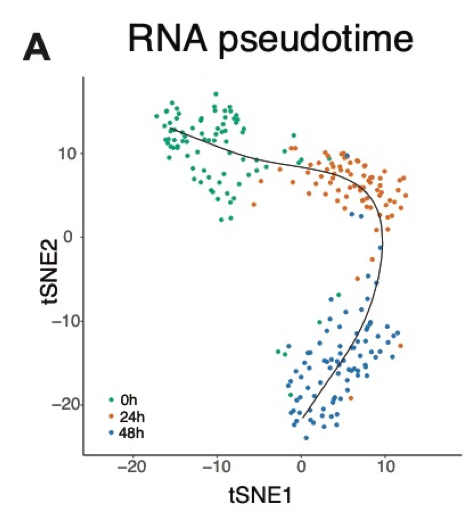] --- name: SPARC2 class: spaced ## SPARC - Single-cell Protein and RNA Co-profiling .size-100[] .pull-left-70[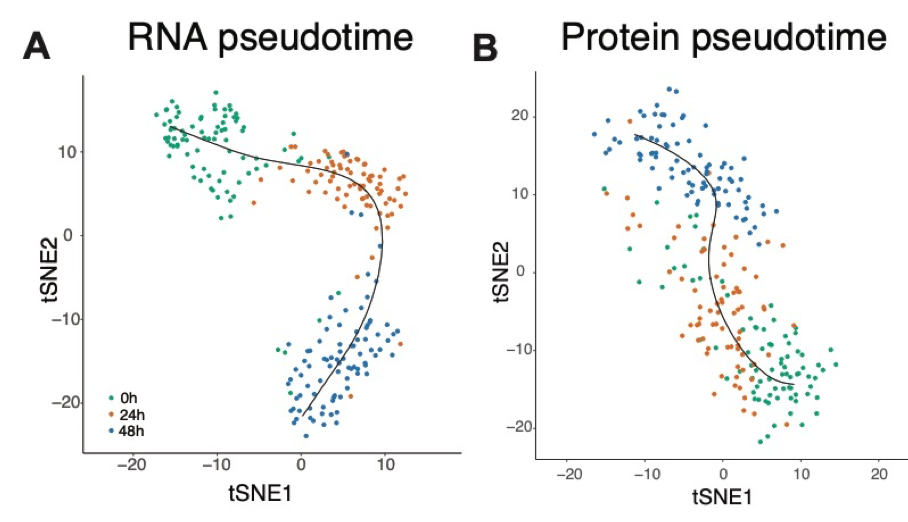] --- name: SPARC3 class: spaced ## SPARC - Single-cell Protein and RNA Co-profiling .size-100[] .size-100[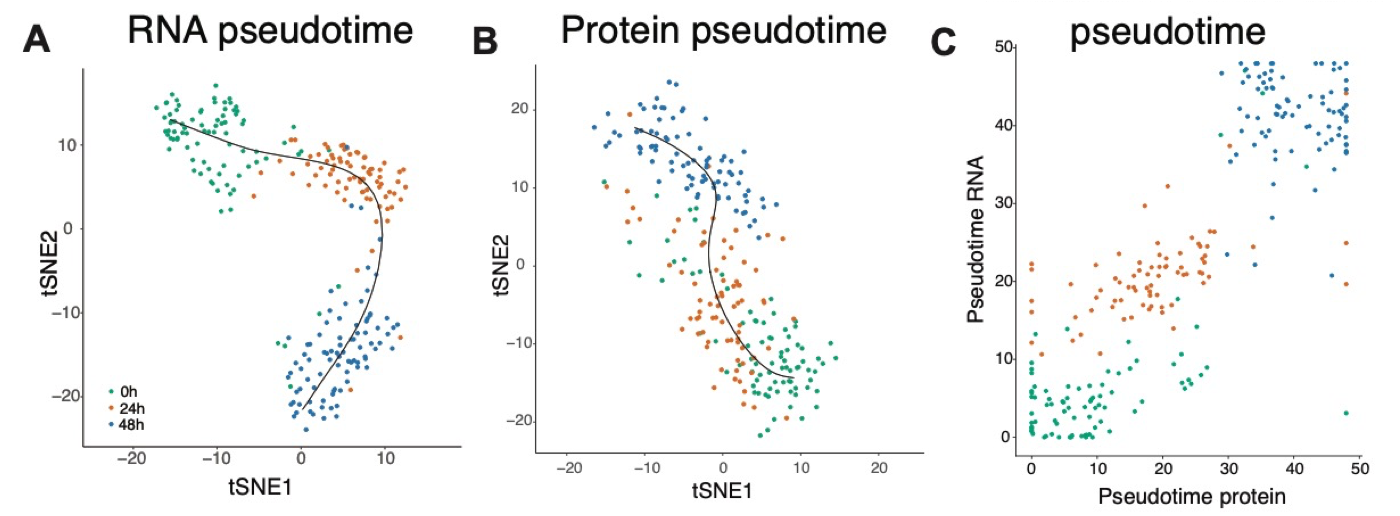] Pearson correlation between RNA-protein pseudo time: 0.83 --- name: SPARC3 class: spaced ## Correlation of mRNA and protein Using our data mRNA expression fails to accurately reflect protein abundance at the time of measurement .pull-left-25[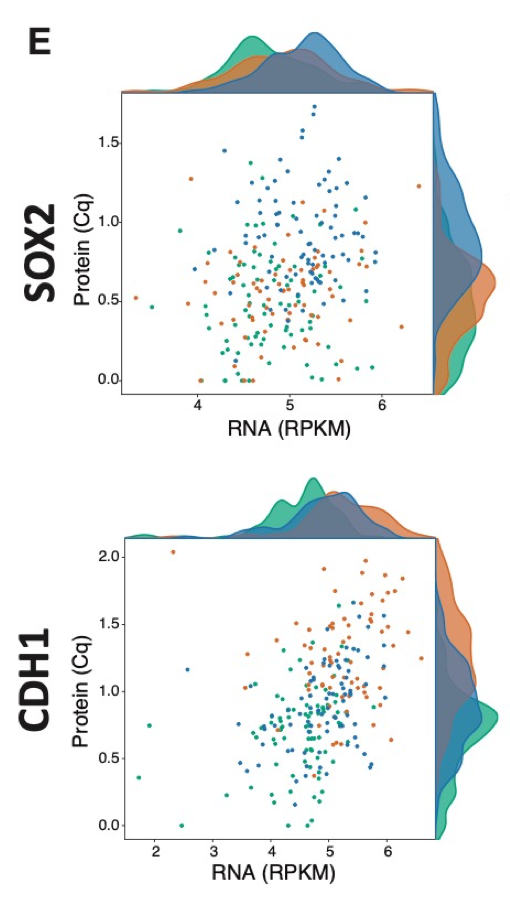] --- name: SPARC3 class: spaced ## Correlation of mRNA and protein Using our data mRNA expression fails to accurately reflect protein abundance at the time of measurement .pull-left-50[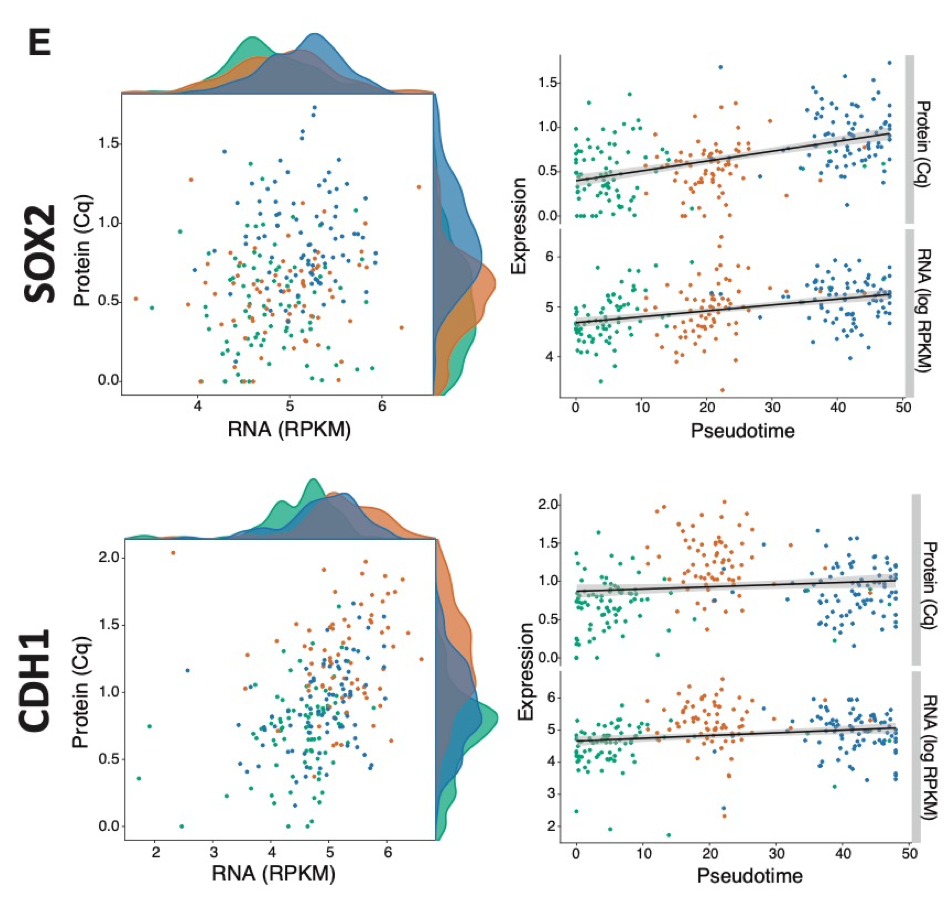] --- name: SPARC3 class: spaced ## Correlation of mRNA and protein Using our data mRNA expression fails to accurately reflect protein abundance at the time of measurement .size-100[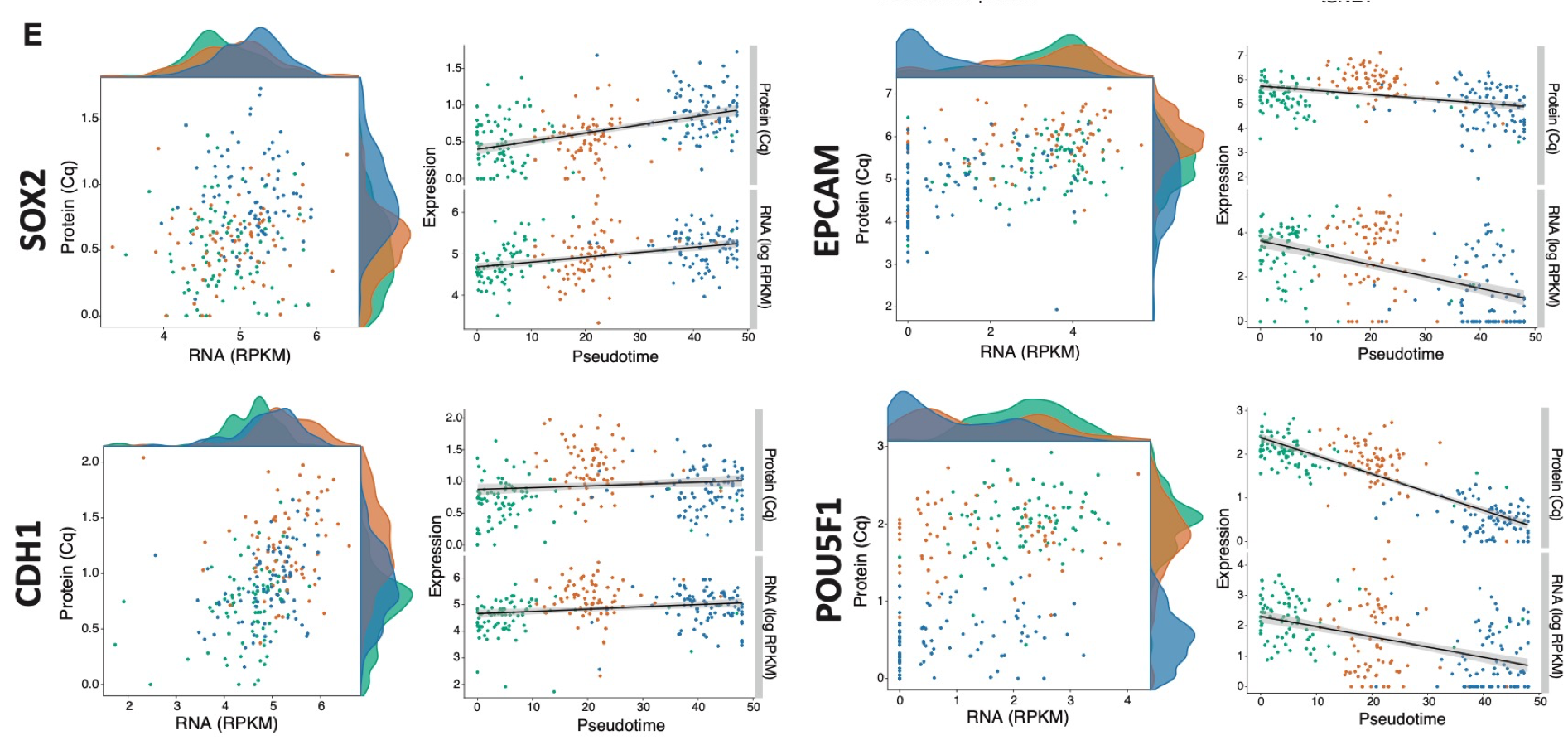] --- name: SPARC3 class: spaced ## Correlation of mRNA and protein Using our data mRNA expression fails to accurately reflect protein abundance at the time of measurement .size-80[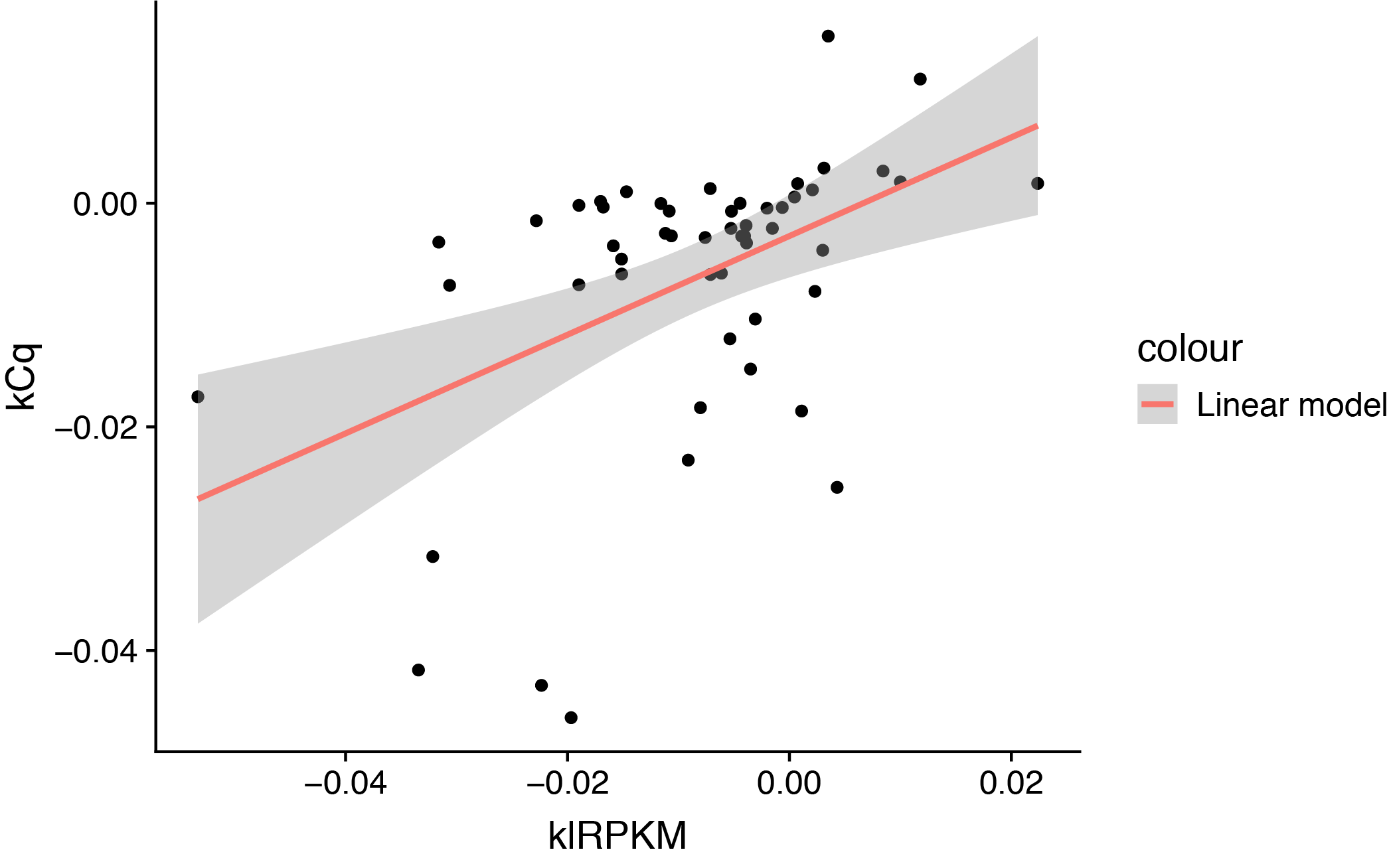] - A weak, but significant, trend that RNA and protein levels correlate over time. - Very seldom contradictory results, *e.g.* protein levels go up and RNA levels go down, over time. --- name: Velocyto class: spaced ## Can we use mature RNA and protein levels to predict the flow of cells over time. #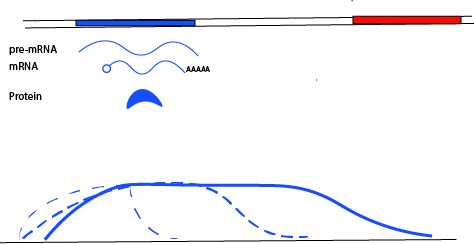 Can we use velocyto and replace pre-mRNA levels with mRNA levels and mRNA levels with protein levels --- name: SPARC3 class: spaced ## Can we use mature RNA and protein levels to predict the flow of cells over time. #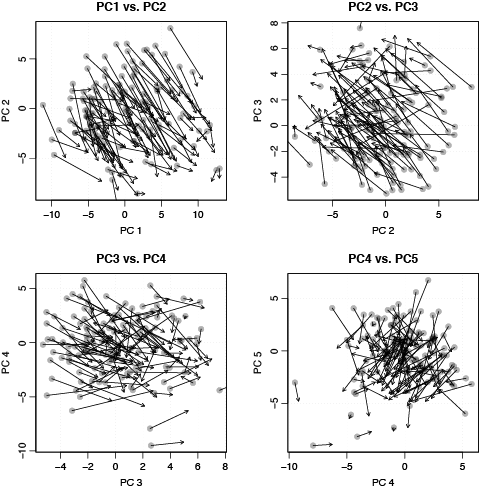 NO! --- name: SPARC3 class: spaced ## Can we use mature RNA and protein levels to predict the flow of cells over time. #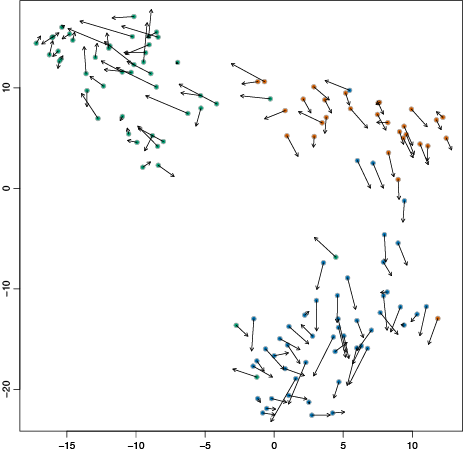 MAYBE... --- name: SPARC3 class: spaced ## Are protein level of TF better to predict TF-targets? #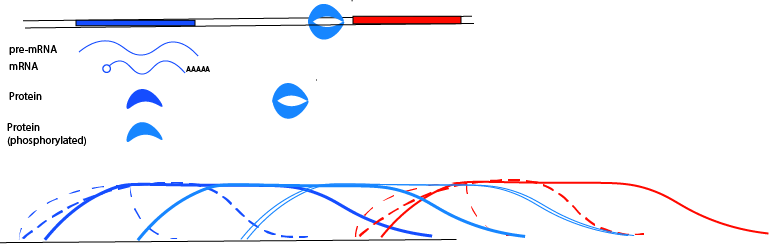 --- name: SPARC3 class: spaced ## Are protein level of TF better to predict TF-targets? .size-60[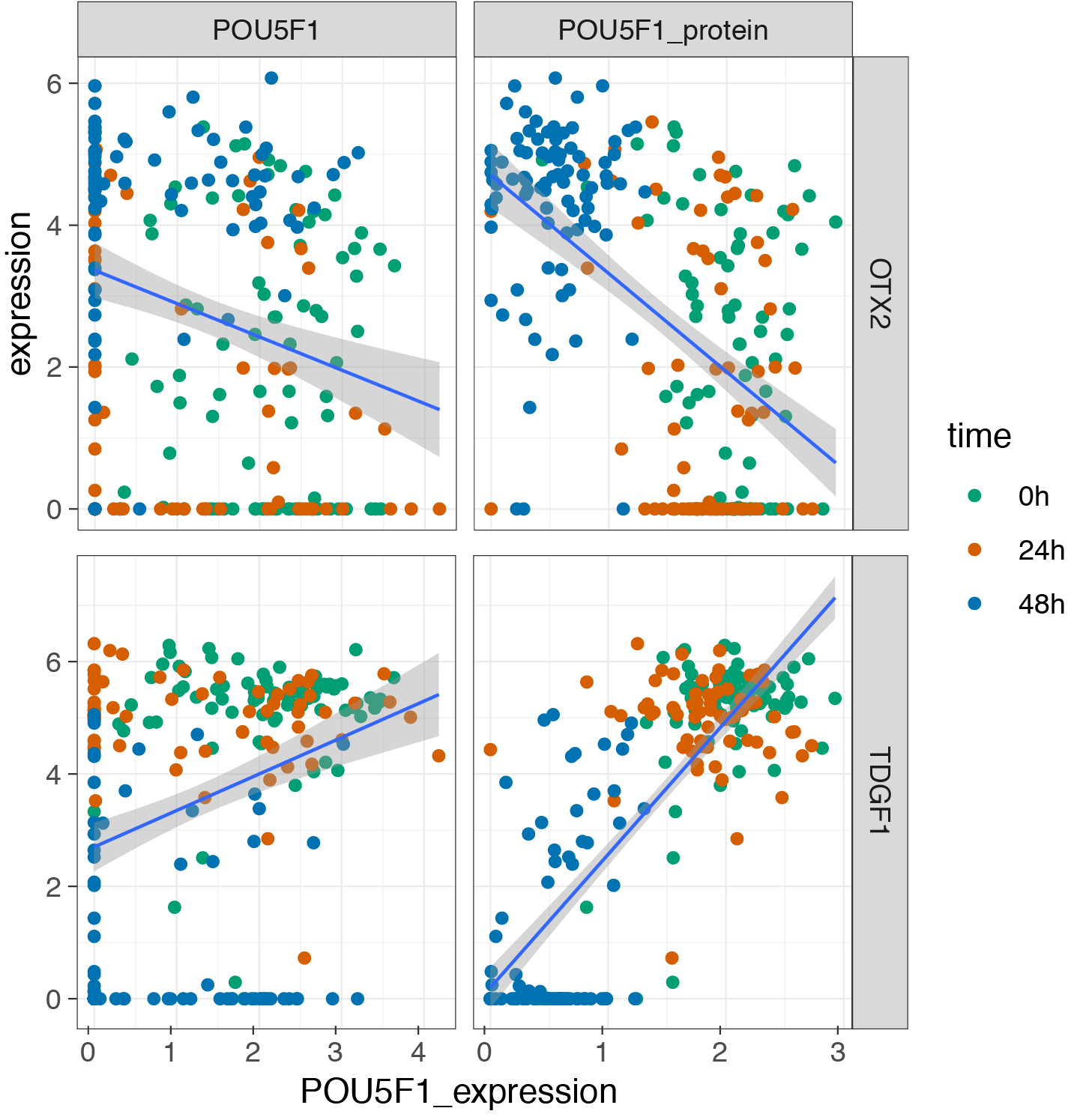] * Yes. at least for POU5F1 --- name: SPARC3 class: spaced ## Are protein level of TF better to predict TF-targets? .size-60[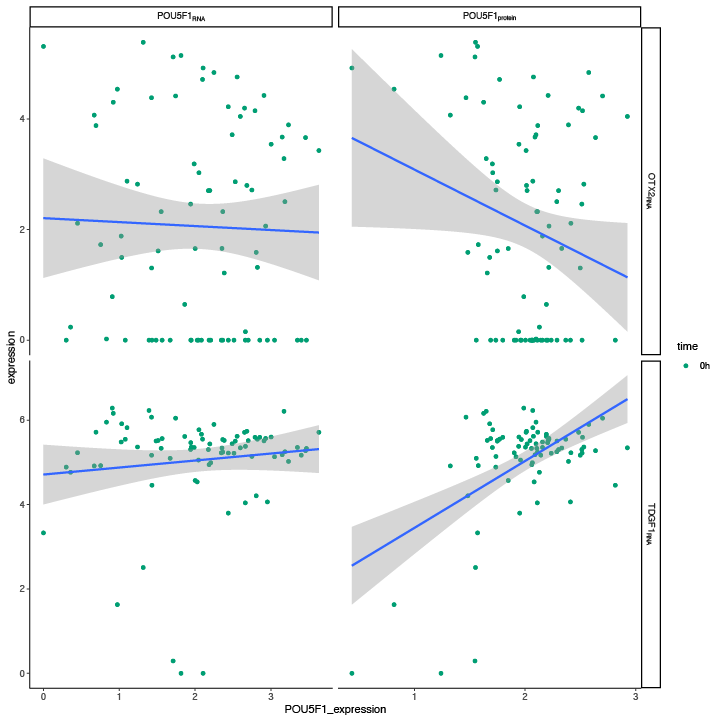] * Signal remains for single cells in 0h steady state for protein but not for RNA levels of POU5F1 --- name: SPARC3 class: spaced # Are protein level of TF better to predict TF-targets? .size-100[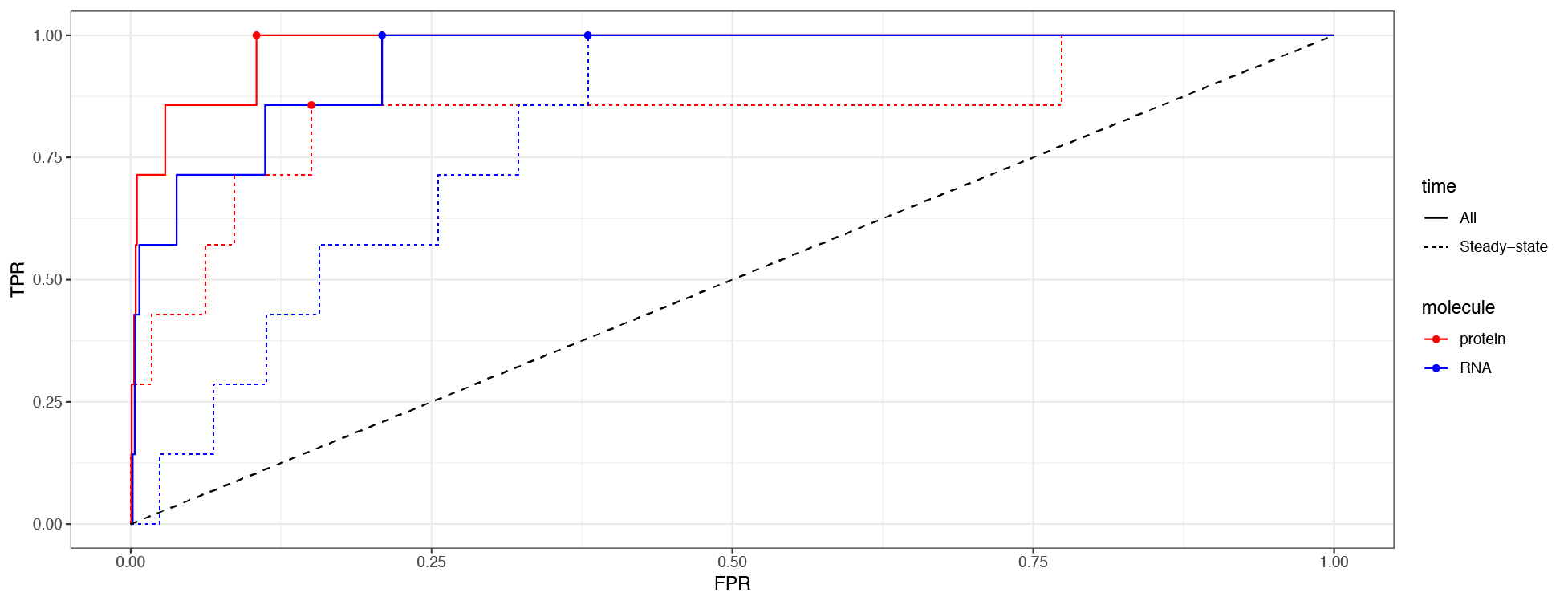] * Protein levels better predictor. * Better predictor if TF changes over time but works also in steady state cells! --- name: SPARC3 class: spaced # Potential POU5F1 targets .pull-left-50[ - Criteria for being a target - Among top correlated levels to POU5F1 in two of the setups - ChIP seq data evidence that POU5F1 binds in the vicinity of the TSS of the gene - All targets were the same for All time points independent of method - 13 of 20 where also found in steady state samples when using protein level of POU5F1 - 2 of 20 where also found in steady state samples when using protein level of POU5F1 ] .pull-right-40[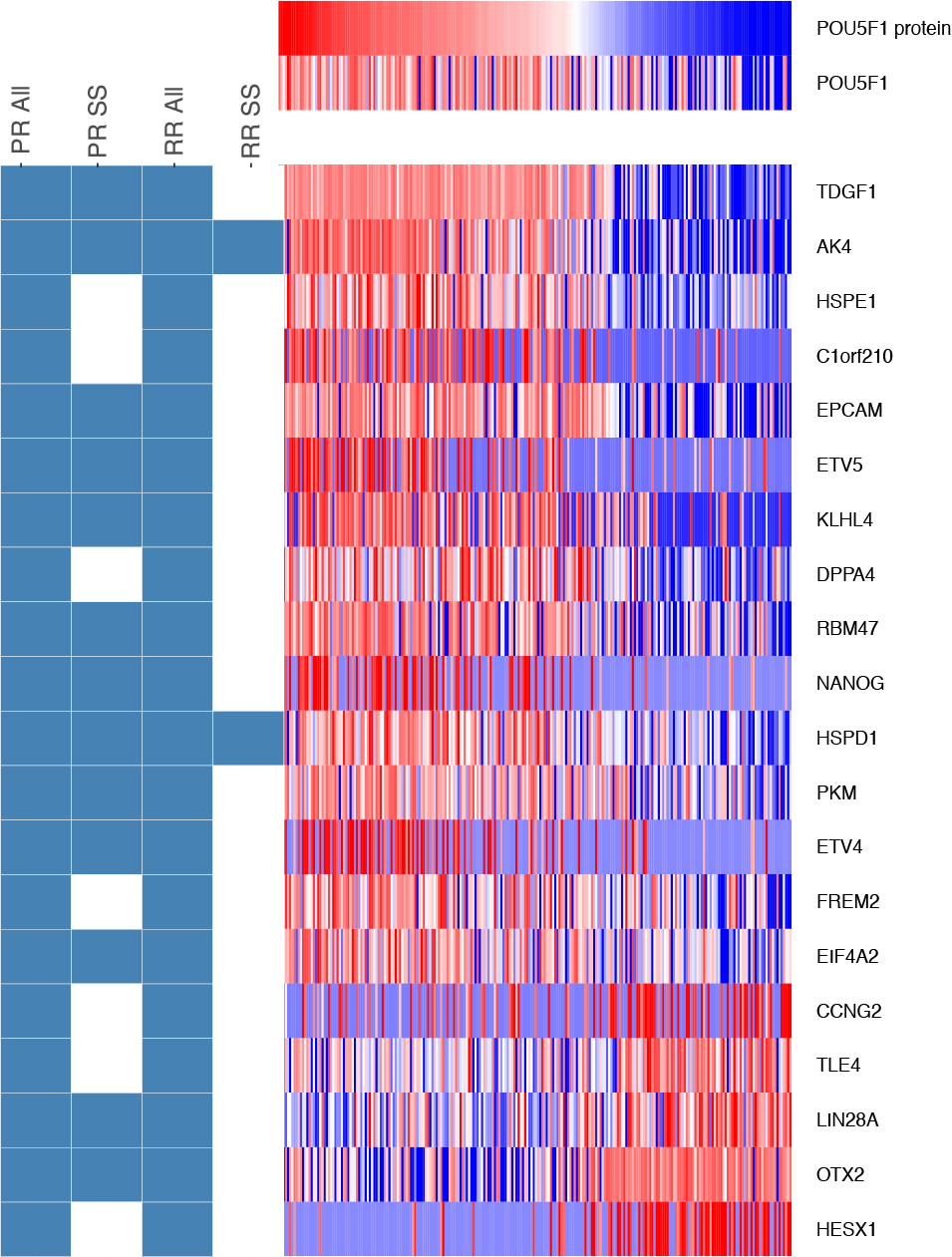 ] .size-40[![]()] --- name: SPARC1 class: spaced ## Single cell mRNA and protein analysis in a dynamic cellular system .padding-horisontal-70[ - Can we co-measure global mRNA and intracellular protein in single cells? - Yes we can! - How are mRNA-protein co-expressed? In cells at steady-state or undergoing a stable-state change? - Very little correlation unless you follow over time or in different cell types. - How can protein measurements aid to decipher cell state and gene regulatory networks? - More stable expression levels ( Not shown in this lecture). Better bio markers. But not as good as the surface protein makers. - Better predictor for TF targets ( at least for POU5F1) ] --- name: SPARC1 class: spaced ## Afternoon lab .padding-horisontal-70[ - Use Seurat and CITE seq data to combine protein and RNA levels to identify different clusters - Use scripts and SPARC data to go through some of the analysis presented today ]